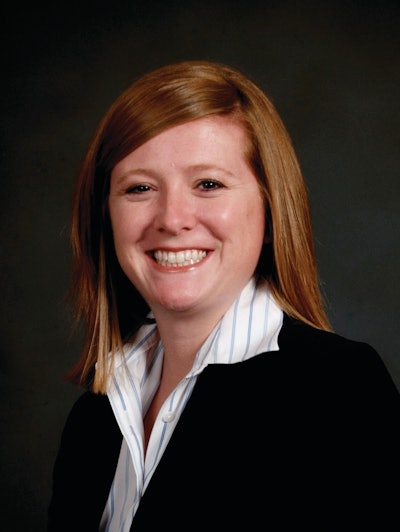
For food & beverage manufacturers, diversifying product lines to capture market share in consumer micro-niches can be a double-edged sword. Multiple product lines can help grow revenue and margins, but it creates challenges for operations. Each new product line brings a new set of recipes, regulations, quality regimens and optimization challenges to the manufacturing plant. In the face of increasing consumer and retailer concerns, manufacturers cannot be driven by choosing between cost and quality—they must have comprehensive operational strategies that address both.
As labor and capital prices in developing countries rise and new FSMA regulations strain imports, supply chain investments of the last decade are in need of upgrades. Through the 1990s and the early parts of the 21st century, food & beverage manufacturers focused much of their attention on external capabilities such as contract production and sourcing; however, the next source of strategic advantages will be found within manufacturing facilities themselves.
The parallel initiatives of improving profit margins and quality commonly compete for financial and human resources, resulting in less effective outcomes on both fronts. The net result is that manufacturers carry higher costs of production through their entire supply chain, while increasing their risk exposure. By more effectively deploying IT systems, manufacturers can bridge the gaps between risk management and continuous improvement programs by using data collection and analysis software.
High Quality/Product Safety Initiatives
In general, quality and safety regimens are concerned with capturing and correlating the following types of data:
Reference Data
• Execution Recipes
• Bills of Material
• Quality Specifications
Operating Data
• Raw material characteristic and quality information
• Recipe execution data (adherence to setpoints, processing times, etc.)
• Consumption/Genealogy data
• Process parameter data (temperatures, speeds, pH reading, viscosity, etc.)
• Line-side quality test data
• Off-line quality test data
• Relevant environmental data (ambient temperatures, humidity, air pressure)
• Cleaning process data (temperatures, durations, etc.)
• Other counts, confirmations and checks (equipment checks, area inspections and so on)
Correlating this data to production lots and to production lines creates the record set that eases decision support for final product release, smooths customer or regulator auditing and also supports root cause analysis when quality issues occur. However, these quality assurance tools are generally applied primarily to prevent release and distribution of at-risk products. If no significant deviation from standards is detected, then deeper scrutiny may not occur. Thus, quality systems are often positioned as “insurance” and perceived as a cost of doing business, rather than a source of insight into opportunity to bolster profit.
Continuous Improvement Initiatives
Continuous improvement programs tend to focus on improving asset utilization, and yields, and will often also be aimed at defining capital improvements that can provide a “structural,” sustainable improvement in output, and/or to incorporate new processing or packaging capabilities.
Common practices require that teams gather, correlate and analyze the following data sets:
Reference Data
• Engineering standards for machine performance
• Execution Recipes
• Bills of Material
• Quality Specifications
Operating Data
• Machine/Asset performance data, including downtime, idle time, changeover time and other non-productive time
• Machine/Asset fault and breakdown data
• Ancillary machine data (oil temperatures, motor loads, etc.)
• Recipe execution data
• Maintenance work order history
Companies that have progressed along their continuous improvement journey will expand this kind of regimen to address the effect of quality on throughput and schedule performance, and will thus analyze additional factors:
• Ingredient/material consumption/genealogy
• Ingredient/material characteristic data
• In-line and offline quality test results
• Process parameter data
• Relevant environmental data
• Effective production rates (as opposed to modeled rates)
Overlap of Separate Initiatives
Looking at the data elements with which each type of program is concerned, and also identifying the sources of information each program relies on highlights the significant overlap between these separate initiatives for quality and improvement.
Most of the differences between the groups’ usage of data are in the area of analytics — how different elements can be correlated and compared, and what boundaries or filters can be placed on different data sets to isolate blocks of data.
From an information perspective, quality/ safety data and continuous improvement data are really two sides of the same coin — manufacturing data.
A Single-Platform Approach to Manufacturing
Data Collection
This is the area where properly architected technology support can really bring both worlds together. To create rich data sets, it is necessary to incorporate as much process and asset data as possible into the regimens. While automation data is readily available via Ole for Process Control (OPC) or other technologies, to be useful, the data extracted must be contextualized to the same “markers” as non-automation data – orders/lots, assets, process stages, specific quality or safety checks, etc.
This is, in fact, one of the areas where quality and continuous improvement programs have diverged in the past. Quality systems have been focused so much on specific, relatively infrequent (minutes or hours vs. sub-second) events such as quality tests or logging of ingredient consumption, that many of them have been deployed in fairly traditional operator-entry styles.
Continuous improvement programs aimed at asset utilization or process tuning have tended to focus on large sets of non-contextualized machine data, looking for trends or patterns independently of “markers” such as materials in process or product types.
While recent trends in OEE analysis and yield analysis have driven engineering teams to include more contextual data in their analyses, the reverse is not as true for the adoption of rich automation-based data into quality and safety regimens. As a result, a quality issue may lead to a uniquely designed investigation that must be re-invented when the next risk is recognized, and the next, and the next and the next…
Modern manufacturing IT foundations combine both event-based data, recorded into relational database systems, with high volumes of granular time-series data collected from automation sources in order to support data collection and reporting regimens for both groups of stakeholders. By automating the reporting regimens used for daily performance monitoring as well as for deeper analysis, such platforms ease the investigative processes that are triggered by exceptions in relation to quality or to process performance.
Reporting and Correlation
Extending the contextualization of the core models to automation data provides the basis for a truly powerful correlation capability that enables a manufacturer to extend the analysis of product risk or throughput losses across a number of dimensions.
Such multidimensional analysis is eased when supported by a detailed process and event model; the model creates additional markers that can be used to delineate the boundaries of different inquires. It is in this area that the specific forms or tools applied tend to be similar between the quality and improvement teams. Each will begin its work by assessing Key Performance Indicators (KPIs) and drill through a mix of structured analytical tools such as SPC charts as well as relatively unstructured trend and event logs. Differences tend only to be found in what data is driven into a particular chart or view. Again, the correct approach is to provide an IT platform that provides common tools, with the flexibility to populate them according to the immediate needs of a given user.
Sustaining Gains Over Time
Beyond the simple consideration of easing deployment, the common platform creates additional value:
• A single platform as the source of manufacturing data eliminates doubts about corrective action or remediation activities that are caused when multiple stakeholders present conflicting views of an issue.
• The common toolset makes cross-pollination of reports and analysis easier. As quality and improvement teams develop a deeper understanding of the interrelationships between the factors they analyze separately, the common framework will foster cross-functional collaboration, and development of improved work processes and related analytical support.
Conclusion
Food & beverage companies’ product diversification strategies create opposing forces that act on profits and potentially on brand equity. While market share and profit margins are both reinforced, the rapid pace of product launches can reduce operating efficiencies. At the same time, the explosion of new recipes and specifications raises the difficulty of maintaining and providing product quality and safety. Traditional practices around quality and continuous improvement programs have led to internal competition for investment in supporting technologies, but there is significant overlap between these stakeholders with respects to data collection and analytics.
A better approach to supporting both sets of stakeholders is to develop work processes that incorporate data collection for both sets of requirements, and to supplement those work processes with systems that reduce the intrusiveness of data collection on production workers. With a robust set of data available, addressing the specific needs of risk management teams and continuous improvement teams becomes a matter of tailoring information integration and reporting to support the specific requirements of these critical functions.
Strategically, this approach equips a food & beverage manufacturer to accelerate new product launch cycles, knowing that manufacturing is equipped with the tools to manage safe, profitable high-mix production.