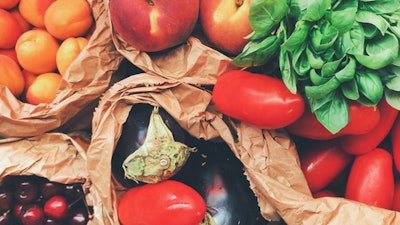
Fresh produce is a critical ingredient for food companies that make juices, sauces, pulp, paste, smoothies, etc. Here, fresh produce pertains to both fruits and vegetables.
Their characteristics vary by variety and season, which creates challenges in the manufacturing process.
Measuring quality in fresh produce
Defining fresh produce quality parameters and their measurement for acceptance is more complex compared to other raw ingredients. Uniformity, ripeness, color, gloss and absence of defects are just some of the components of quality. Evaluating them can be subjective (manually) or objective (machine).
But, the visual appearance of fruits and vegetables is universally accepted as the first quality determinant. Color is an important parameter because it directly affects the appearance of the final product.
If there is color variance in fresh produce inputs, corrective actions in the manufacturing process become necessary to ensure the quality of the final product.
In short, failure to meet fresh produce color criteria can adversely impact the cost, quality or both. This applies to a wide range of food industry products.
Obstacles to identifying color
The biggest challenge to determining the color of fresh produce is heterogeneity. It means that the color of the fruit or vegetable may not be one color, but different shades of the same color. It could also be different colors blending into one another.
A good example is tomatoes. A single tomato may have red, orange and light green colors and still be acceptable as an ingredient. Another example is apples, which may have different shades of red depending on the variety.
Traditional solutions for identifying color
The color heterogeneity in fresh produce makes it difficult to set and measure acceptable criteria. Right now, the food industry utilizes a battery of equipment to measure color in both ingredients and finished products. Most often, they are colorimeters, spectrophotometers and image-based color identification machines.
Though made for myriads of food (and non-food) ingredients, traditional instruments mostly fail in measuring acceptable color criteria for fresh produce.
· Colorimeters are best for gauging homogeneous solutions or solids. When faced with heterogeneous solids, they at best provide an average color. It often results in inaccurate identification.
· Spectrophotometers are most appropriate for input in a liquid medium and homogeneous products.
· Equipment that process high-quality images in a controlled environment appears to be the ideal choice. At least, among existing solutions for fresh produce. These go beyond average color measurement to offering percentage composition of colors.
For example, in the case of tomatoes, they can detect that the displayed tomato is 80% red and 20% light green. But, when you show five tomatoes together, where one is light green and four red, they fail. Since the combined composition is still 80% red and 20% light green, it would lead to the one light green tomato getting accepted erroneously.
Innovative solutions for identifying color
In the last few years, artificial intelligence (AI) has widened the lead over prior technologies for accuracy in visual identification of fresh produce. It also excels at image processing, and beaten previous benchmarks in object detection, classification and segmentation.
To explain these tasks, let’s take up the example of tomatoes again:
· Object detection is the ability to identify different objects in an image. In this case, all the individual tomatoes presented.
· Classification is the ability to organize an image into one of many classes. In this instance, the classification of a tomato can be as 80% red or 30% red.
· Segmentation is the ability to distinguish the composition of an image into different features that are present in it. In this example, tomatoes, table, piece of paper, etc.
Two factors enable AI to achieve excellence in these tasks. One, a collection of a large dataset of images labeled to meet acceptable and non-acceptable parameters of fresh produce. Two, sizable amounts of computing ability, i.e., powerful microprocessors.
In a nutshell, AI fixes the issues riddling color for fresh produce accurately and much better than ever before.
Advantages of AI in identifying color
AI-based solutions have the unerring ability to eliminate color issues in a heterogeneous scenario and color acceptance criteria for almost all fresh produce. But, that’s not the sole benefit. It can do so in a wide variety of lighting.
On top of it, with cloud processing, the solution becomes highly portable. It means one can conveniently access the powerful technology through a handheld device like a mobile phone.
Also, cloud computing allows easy integration of the solution with the rest of the user’s applications such as mail and messaging. These qualities make AI-based solutions far lighter on a user's pocket than the bulky, traditional equipment used for color identification.