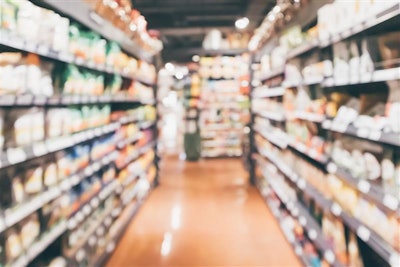
The consumer packaged goods (CPG) industry has experienced dramatic swings in supply and demand in recent years. From fluctuations in consumer spending, shifting consumer demands, shortages of raw and processed ingredients and external supply chain disruptions caused by natural weather events and geopolitical unrest, CPG manufacturers have been forced to navigate a seemingly unending obstacle course.
If manufacturers are struggling to analyze challenges and identify specifically how those challenges will impact operations, they need digital tools that enable predictability and agility. Digital manufacturing strategies, which incorporate the convergence of information technology (IT) and operational technology (OT), enable manufacturers to increase agility by determining the best course of action more efficiently. After all, the best way to make it through an obstacle course is with agility to be ready for the next challenge.
Understanding and preparing for AI-driven optimization
Automation and intelligent analysis, supported by artificial intelligence (AI) and machine learning (ML), are two of the core capabilities that enable a smart manufacturing approach to business management. Predictive AI offers nearly countless benefits to manufacturers that effectively execute digital transformation. However, if the goal is to optimize operations by leveraging AI technology, effective execution of digital transformation can be a tricky process, especially for manufacturers that don’t have the resources to hire a data scientist.
Data preparation continues to be one of the biggest challenges in preparing an organization’s IT and OT landscapes to use AI-/ML-driven tools. It’s easy to understand why: poor data quality leads to incorrect predictions. If you’re trying to solve a math problem but you put the wrong numbers into a calculator, you wouldn’t expect it to give you the correct answer to the problem. AI tools can be viewed as just that: a calculator; albeit a very powerful calculator.
Enabling IT/OT convergence for predictive AI
For manufacturers, incorrect predictive data analysis leads to faulty conclusions that negatively impact production, worker safety and capital-intensive equipment. Manufacturers need to feed comprehensive, quality operations technology (OT) data into IT systems to drive predictive AI analysis and avoid these negative impacts. Automating the capture of OT data in real-time at the edge, directly feeds quality information to IT systems for AI-driven analysis and eliminates the need for heavy data engineering efforts when analysis is needed.
Most manufacturers don’t want, or need, to invest in heavy data engineering efforts to take advantage of predictive AI and accelerate time-to-value. Pre-built libraries of AI and ML algorithms, designed for specific manufacturing use cases, enable OT engineers and IT analysts to speed the deployment and use of predictive AI.
Rich OT context, collected from historic production and machine data, enables CPG manufacturers to identify enterprise-level insights. These insights inform sourcing and production strategies and accelerate time-to-value. For example, if a manufacturer expects a sudden increase in demand for a specific product, they can use OT data, along with inventory and procurement information, to fuel AI analysis and determine how sourcing and production strategies should be altered to meet demand based on historic performance.
Empowering workers with visualized predictive AI model performance
The digital transformation journey to predictive AI isn’t quite over yet. Manufacturers can’t just sit back and expect ML algorithms to do everything without supervision once predictive AI is enabled. Supervision through visualization is the key to managing ML algorithms throughout the model’s lifecycle in a centralized IT environment. Visual representations of performance make supervision of the algorithm more intuitive by enabling an easy understanding of model accuracy.
After creating an analytics model, manufacturing IT teams must track and document the performance of the ML model. In addition, just as a good employee requires continuing education to learn new skills, AI and ML models must be regularly retrained with new OT production data. Continuously retraining the model is necessary to overcome the challenges that drive manufacturers to adopt predictive AI in the first place: rapid change. Market conditions inevitably change -- new shop floor machinery assets and approaches to manufacturing are constantly being developed and customer demands shift.
Manufacturers should seek solutions that empower them to visually build, train, deploy, score and monitor ML models on an ongoing basis. Visualizing transformed data throughout the analysis process minimizes unexpected errors found in the final insights. This enables IT analysts to ensure accuracy and adjust models as needed.
Understanding the steps to enabling AI analysis
Some manufacturers are already preparing for, or deploying, AI-driven solutions to improve their agility. For example, a large European dairy producer was struggling to meet regulatory standards surrounding the drying process of caseinate powder, which required dryers to run slower to avoid waste caused by noncompliance. In turn, this caused the dryers to consume more energy, as well as a reduced throughput. The dairy producer has successfully leveraged OT sensors, paired with IT-driven predictive models, to increase throughput and maximize resource efficiency. By utilizing sensor data, model-based closed-loop controls rapidly responded and adjusted dryer settings when the IT system predicted any issues with moisture, throughput or energy consumption. Through the integration of OT and IT technology, the dairy producer achieved a 42% reduction in quality variability, which resulted in up to a nearly 16% increase in throughput.
The exact use of AI-driven analysis will vary from facility to facility. CPG manufacturers seeking to take the first step toward enabling AI analysis should consider their facility’s various stages of production (preparing or cooking ingredients, filling, bottling, packaging, etc.). Next, they should explore where and how context data provided by OT will have the biggest impact on desired outcomes (improved productivity, reduced waste, etc.) throughout the facility and production process. Finally, a visually-oriented data science tool must be deployed to analyze the OT data. This will enable the manufacturer to cleanse the data and perform root cause analysis of production process challenges in real-time, providing the opportunity to identify and rectify the exact issues that impede the achievement of desired outcomes.
A predictable yet exciting future
AI for industrial purposes is changing the way manufacturers approach, think about and execute business operations – not only in the boardroom but across the enterprise. Incorporating AI into regular facility workflows has the potential to improve nearly every aspect of a manufacturing business, from procurement and sourcing practices to production scheduling as well as a host of other critical functions.
As much as AI analyzes information to identify the best course of action, manufacturing IT and corporate leaders must analyze the changes taking place in the manufacturing industry and identify the best digital transformation strategy. Manufacturers that approach transformation in an intelligent way, by knowing exactly how to execute digitalization and the impacts of transformation, will be best equipped to predict the next business challenge, meaning they will be the first to overcome it – establishing competitive differentiation and thriving in a seemingly unpredictable market.